In today's hyper-competitive business environment, customer support has become a key differentiator for companies looking to stand out. Before you can better cater to your shoppers' needs, you must know what they are and how they might change. Collecting and analyzing data can quickly become daunting, particularly when dealing with large volumes of customer information.
Fortunately, predictive analytics has emerged as a powerful tool for enhancing customer support by allowing companies to anticipate customer needs and provide personalized, proactive support.
What is predictive analytics in customer support?
When we say predictive analytics, we’re talking about collecting and using data to predict future trends and events.
This can be done in a variety of different ways. In this blog, we'll explore 5 real-life examples of businesses that successfully leveraged predictive analytics to enhance customer support and improve the overall customer experience.
5 Ways to Use Predictive Analytics in Customer Support [From the Businesses Who Successfully Did It]
1. Identifying potential issues
In customer service, predictive analytics can identify potential issues by analyzing various data sources. Examples included:
- Collecting customer feedback from a formal survey
- Reviewing service tickets for complaints
- Monitoring social media interactions
By analyzing this data, predictive models can anticipate potential issues that customers may encounter, such as product defects, shipping delays, or service disruptions.
For example, suppose a customer posts a complaint on social media about a delay in getting their order. In this case, predictive analytics can be leveraged to analyze the post's sentiment and identify if other customers are experiencing similar issues. You can then use this information to proactively address the issue by communicating with affected customers or even issuing a general statement.
Use case:
How Coca-Cola uses predictive analytics to identify potential issues

Beverage giant Coca-Cola uses predictive analytics to identify potential supply chain disruptions that could impact its production and distribution processes. By analyzing weather patterns, transportation routes, and inventory levels, Coca-Cola can predict when a particular region may experience supply chain disruptions and take proactive steps to mitigate the impact, such as moving inventory to other locations, adjusting production schedules, or making alternative transportation arrangements.
2. Personalization at scale
Predictive analytics can be used to personalize the customer experience by analyzing customer data to gain insights into their preferences, behaviors, and needs. By truly understanding each shopper, businesses can create personalized experiences at scale.
For example, companies can use predictive analytics to analyze a shopper’s purchase history – and even pair it with their current browsing behavior – to anticipate their needs and make unique product recommendations.
These recommendations can then be delivered in a couple of different ways:
- AI-powered chatbots can be programmed to proactively launch conversations with prospective buyers. These chatbots can then show a specific item, the entire catalog, or offer to connect shoppers to a product specialist.
- AI-backed support agents can also be on hand or the first to make contact. However, rather than asking agents to memorize product features for an entire catalog, AI can help them quickly match shoppers with the ideal product based on their current and previous shopping history.
Discover how Zowie Grow can help businesses generate customer service-driven revenue.
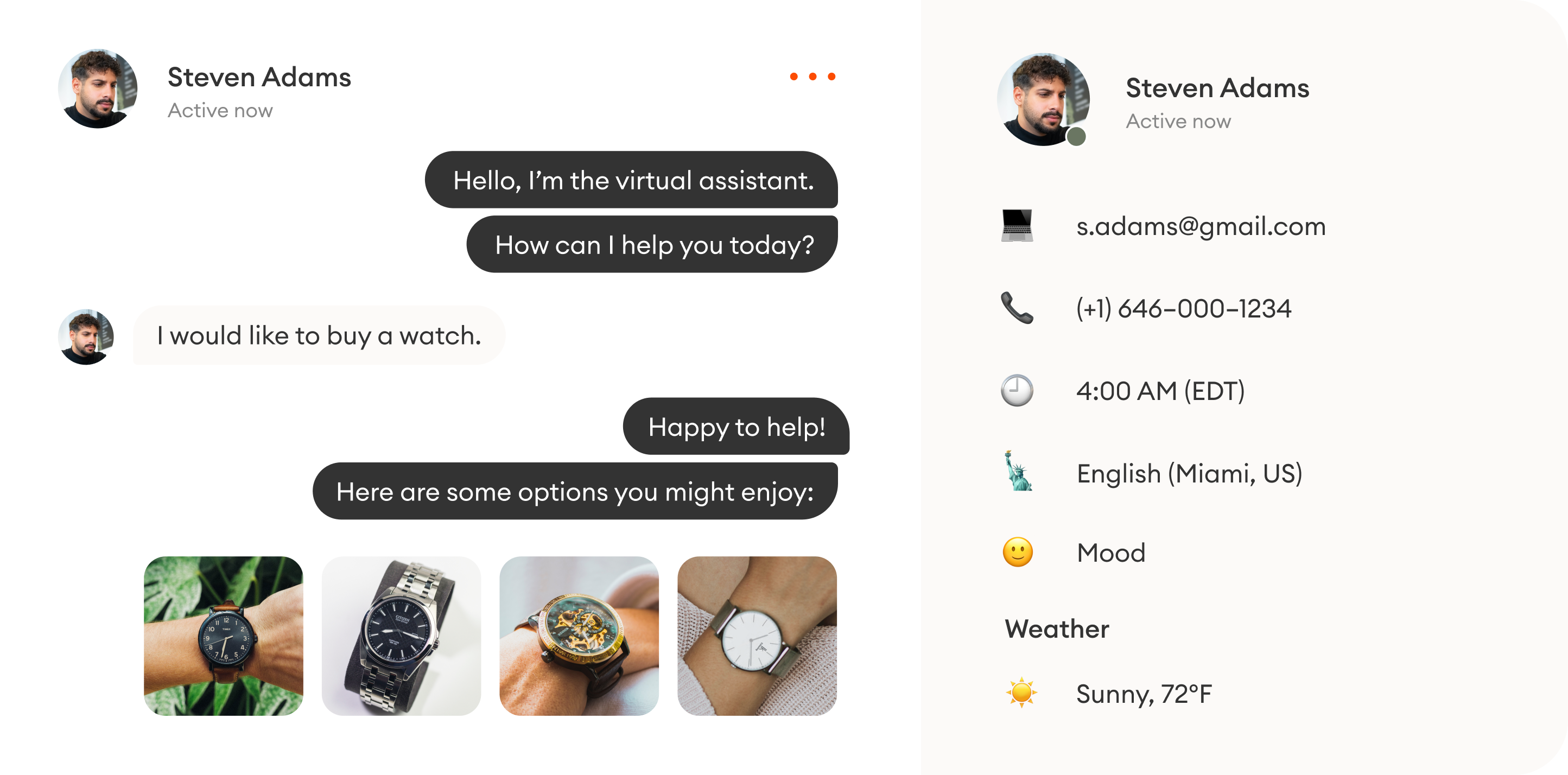
Use case:
How Netflix uses predictive analytics to deliver personalization at scale
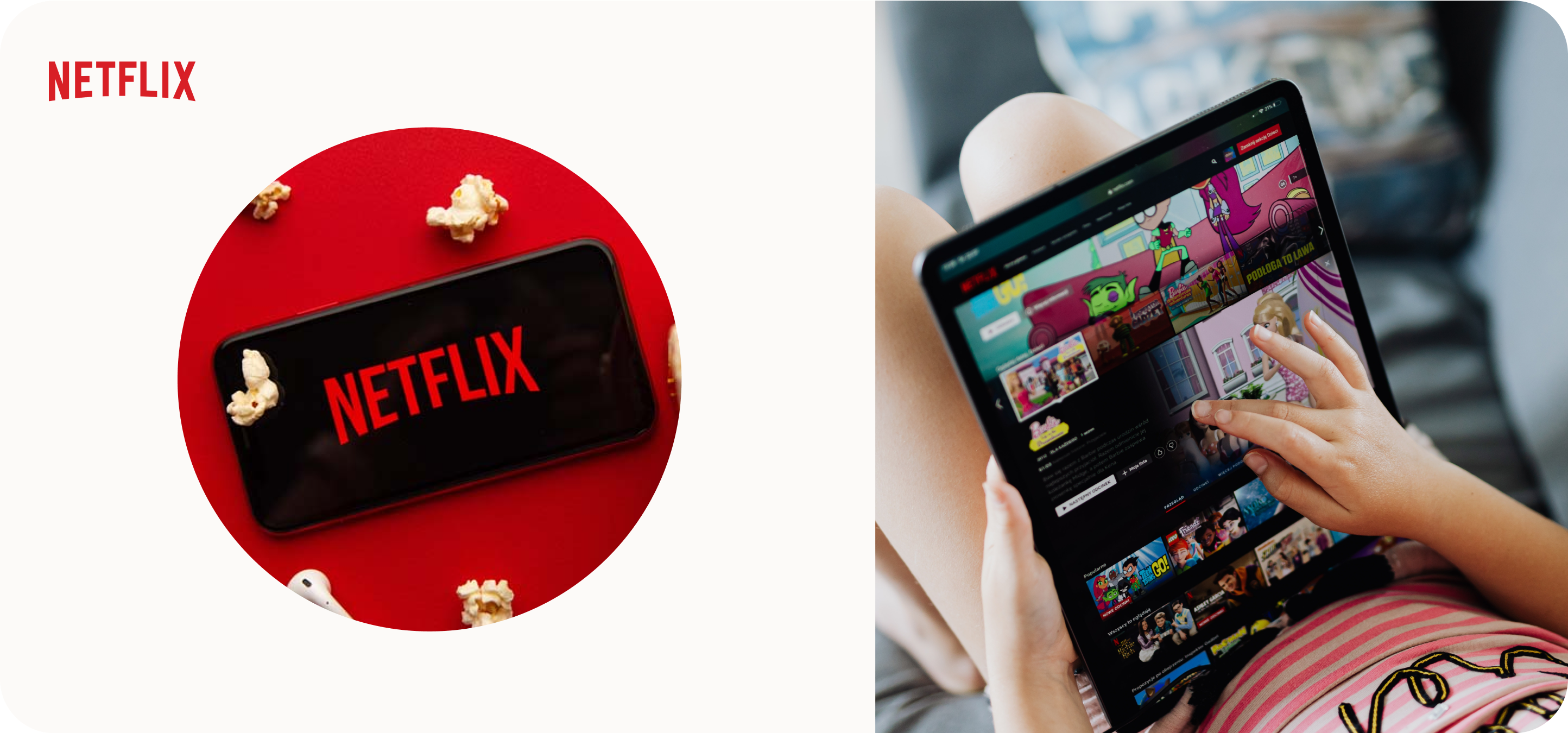
One of the most public examples of this is the famed Netflix algorithm. Netflix collects and analyzes your viewing history, looking for trends in categories such as genres, actors, and directors. It then uses it to search and recommend other shows and movies you may enjoy. This practice of personalization at scale has been a key factor in its success, helping the company to build and maintain a loyal customer base.
3. Improve chatbot accuracy
Predictive analytics can be used to train chatbots to provide more accurate and personalized responses. By analyzing customer inquiries and feedback, businesses can identify common themes or questions and assess the usefulness of their answers. All of which leads to an enhanced customer experience.
At a basic level, this can be done by offering a short survey after every chatbot interaction. Businesses can then see how their chatbot is performing and where improvements should be made. Taking this one step further: leading customer support solutions can provide personalized chatbot recommendations based on incoming questions. This allows you to continuously improve your experience and expand what the chatbot can handle.
Use case:
How Giesswein uses predictive analytics to train and improve their chatbot

Today, European apparel and footwear company Giesswein uses its chatbot to assist with various aspects of the customer journey. Because they previously did not have a chatbot, the program could only handle around 30% of incoming questions when it initially launched. Through AI analysis and customer feedback, that amount jumped to 71% after just two weeks and skyrocketed yet again to 89% within three months.
By providing more accurate customer responses, Giesswein increased satisfaction, loyalty, and even sales.
4. Optimizing resource allocation
Predictive analytics can optimize resource allocation in customer support by analyzing customer demand and service capacity data. This allows businesses to better allocate their resources – like agents – to meet customer demand and minimize wait times.
For example, businesses can use predictive analytics to identify and anticipate high or low-demand periods. Using this information, they can look to allocate more resources during peak hours or seasons to ensure quick and effective service or cut back during "slow" times.
Use case:
How Delta Air Lines uses predictive analytics to optimize their customer support resources
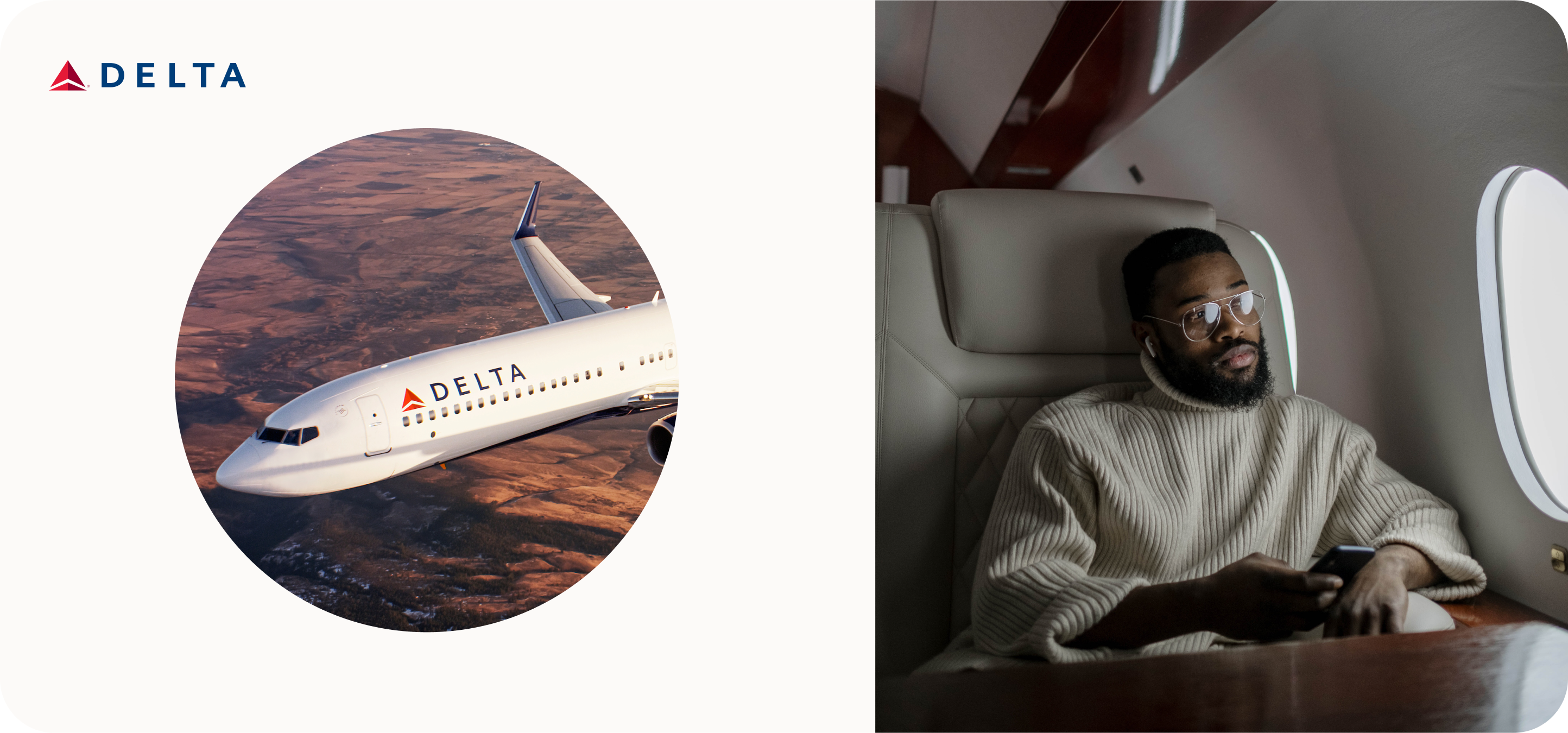
Delta Air Lines uses predictive analytics to forecast customer demand based on flight schedules, historical patterns, and special events. This data predicts how many customer support agents are needed at a given time. Delta can then adjust its staffing levels to avoid overstaffing during periods of low demand and ensure enough agents are available to handle customer inquiries during its busiest periods.
5. Predicting customer behavior
Predictive analytics can be used to analyze customer data, and predict future behavior – such as whether someone is likely to make a purchase or perhaps churn and move to a competitor.
First, it can identify high-value customers and predict their potential lifetime value to the company. By analyzing transaction history, purchase behavior, and customer feedback, companies can pinpoint customers likely to generate the most revenue over time. They can then use this information to prioritize their needs – whether that's offering them the same agent every time they visit the site or in the form of exclusive deals.

In order to predict whether a customer may churn, predictive analytics models can analyze data that tracks the frequency of transactions or a change in browsing behavior. Companies can then use this information to try and re-engage with the individual.
Use case:
How Missouri Star Quilt Co. uses predictive analytics to predict customer behavior
.png)
Quilting leader Missouri Star Quilt Co. has used predictive analytics to better cater to their clientele. Zowie's AI solution has helped them identify which customers on their website are most likely to make a purchase. Using this information, Zowie can automatically offer them personalized product recommendations based on their past purchases or current browsing history. Taking this one step further it can also pass this information onto a live support agent who can step in and deliver white glove service.
This combination helped them achieve a 47% increase in their average order value (AOV) and counting.
Partner with Zowie to unlock the power of predictive analytics in customer support
Want to add predictive analytics to your business plan? With Zowie, businesses can automate routine customer service tasks, leverage customer insights to identify trends and opportunities and provide proactive customer support. Meet with a Zowie rep today and see how our AI can improve customer satisfaction and loyalty, increase revenue, and drive long-term growth.
.png)